Label Images for Object Detection: Unlocking the Power of Data Annotation
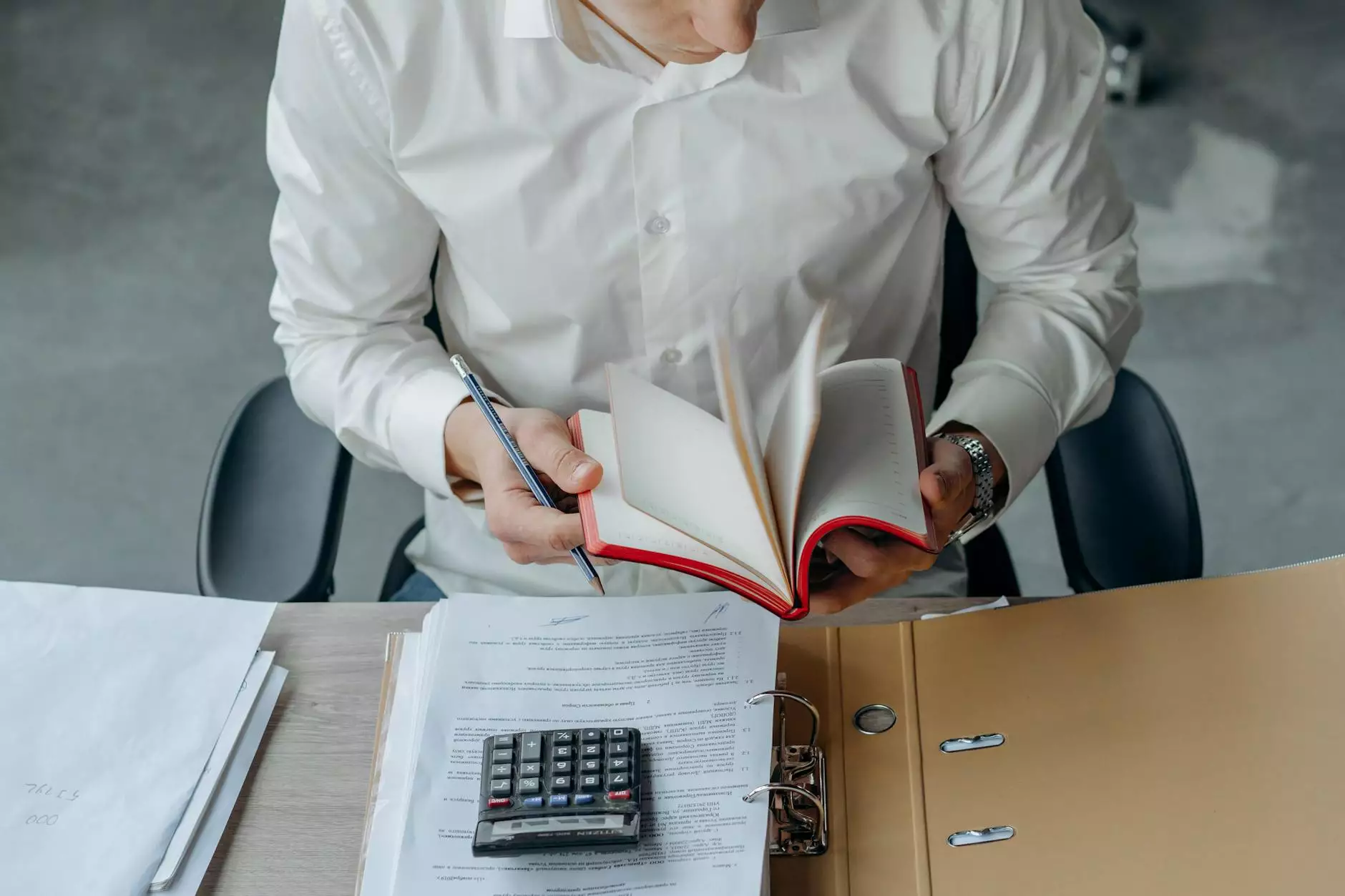
In today’s digital era, the value of data cannot be overstated. As businesses strive to harness the power of artificial intelligence (AI), they are encountering a critical step in the process: labeling images for object detection. This is where data annotation comes into play, serving as the backbone of effective AI training. This article will delve into the importance of data annotation, the role of labeling in object detection, and how platforms like Keylabs.ai are revolutionizing the way businesses approach this essential task.
The Importance of Data Annotation
As businesses invest in AI technology, the need for high-quality data annotation becomes increasingly crucial. Effective data annotation involves annotating data accurately to train machine learning models. Specifically, when it comes to labeling images for object detection, it involves identifying and marking objects within images to enable AI algorithms to learn from these data points.
Understanding Object Detection
Object detection is a critical capability within computer vision that enables machines to detect instances of specific classes of objects (like vehicles, animals, or humans) in images or videos. The success of this process hinges on the quality of the labeled datasets used during the training phase.
Key Benefits of High-Quality Data Annotation
- Increased Accuracy: An accurately labeled dataset improves the precision of machine learning models.
- Time Efficiency: Proper annotation speeds up the training process, allowing for quicker deployment of models.
- Cost Reduction: Reducing model errors through high-quality annotation translates to lower costs in the long-term.
The Process of Labeling Images for Object Detection
The process of labeling images for object detection can be broken down into several key steps:
1. Collecting Data
The first step is gathering a robust dataset that accurately represents the real-world scenarios the AI will encounter. This data can come from various sources such as online repositories, user-generated content, or in-house data collections.
2. Choosing the Right Annotation Tool
Choosing the right data annotation tool is critical. Tools vary in functionality and user interface, so it’s essential to select one that meets your project’s needs. Keylabs.ai provides an efficient platform that supports various annotation tasks, enhancing user experience and productivity.
3. Annotating the Data
This step involves annotators marking objects in images according to defined categories. For object detection, this includes drawing bounding boxes around the objects of interest and labeling them accordingly. Consistency in this step is vital for producing reliable datasets.
4. Quality Assurance
Once the annotation is completed, conducting a quality assurance review is crucial. This ensures that the annotations are accurate and consistent. Feedback loops and verification protocols strengthen the reliability of the datasets.
Best Practices for Labeling Images for Object Detection
To ensure that the object detection models achieve the highest accuracy possible, consider the following best practices:
- Define Clear Guidelines: Have comprehensive guidelines so that the annotators have a clear understanding of how to label objects accurately.
- Use Collaborative Annotation Platforms: Utilizing platforms that support team collaboration can drive efficiencies and foster better communication.
- Implement Active Learning Techniques: Continuous training and feedback for annotators can enhance their skills over time, improving the dataset quality.
- Regularly Update Datasets: As real-world scenarios change, keep your datasets current by revisiting and adding new data.
How Keylabs.ai Elevates Data Annotation
When it comes to labeling images for object detection, Keylabs.ai stands out as a premier data annotation platform that integrates innovative technologies to enhance the data annotation process.
Cutting-Edge Features of Keylabs.ai
1. Intuitive User Interface
The platform offers an intuitive user interface that allows annotators to work efficiently without the steep learning curve. Whether for a beginner or an expert, the interface supports a smooth workflow.
2. Automation Capabilities
Keylabs.ai integrates advanced machine learning algorithms that automate parts of the annotation process, reducing manual work while maximizing output. This allows teams to focus on more complex tasks.
3. Scalability
The platform is designed to scale up with your projects. Whether you have hundreds or thousands of images, Keylabs.ai can handle large project scopes without compromising on quality.
4. Comprehensive Support and Training
With dedicated support and training, Keylabs.ai ensures that your team is well-equipped to maximize the platform's capabilities. Accessing resources, tutorials, and direct support transforms a challenging process into a streamlined operation.
Real-world Applications of Object Detection
Labeling images for object detection opens the pathway to numerous real-world applications. Here are some areas benefitting from this technology:
1. Autonomous Vehicles
In the world of self-driving cars, object detection is indispensable. Vehicles must accurately identify pedestrians, other vehicles, and obstacles in real time to ensure safety and efficiency on the road.
2. Retail and Inventory Management
Object detection enhances the retail shopping experience by facilitating automated checkout processes and inventory management. Retailers can track stock levels and monitor consumer behavior with precision.
3. Healthcare Diagnostics
In healthcare, image recognition plays a significant role in diagnostics. Detecting anomalies in medical imaging can lead to early intervention and better patient outcomes.
4. Security and Surveillance
Using object detection in security systems allows for real-time monitoring and alerts, enhancing the ability to detect unauthorized access or suspicious activities.
The Future of Data Annotation in Object Detection
As technology continues to evolve, so too will the tools and methods used for data annotation. The future holds exciting possibilities for improving how we label images for object detection. Enhanced AI capabilities, such as deep learning algorithms, will automate more aspects of the annotation process, leading to higher efficiency and reduced costs.
Conclusion
In conclusion, labeling images for object detection is a vital process that lays the foundation for successful AI applications across various industries. With powerful platforms like Keylabs.ai, businesses can ensure that their data annotation needs are met with precision, scalability, and efficiency. Embrace the future of data annotation and unlock the full potential of your AI initiatives today!
Get Started with Keylabs.ai
Ready to elevate your data annotation process? Visit Keylabs.ai and discover how we can help you label images for object detection effectively and efficiently!